DFG-Graduiertenkolleg: π³ Parameter Identification – Analysis, Algorithms, Applications
Working Group: | WG Industrial Mathematics |
Leadership: | Prof. Dr. Dr. h.c. Peter Maaß ((0421) 218-63801, E-Mail: pmaass@math.uni-bremen.de ) |
Processor: |
Dr. Tobias Kluth
Sabine Eifeld (E-Mail: eifeld@math.uni-bremen.de ) |
Funding: | DFG |
Project partner: |
Prof. Dr. Armin Lechleiter, Universität Bremen Prof. Dr. Werner Brannath, Kompetenzzentrum für Klinische Studien Bremen Prof. Dr. Christof Büskens, Universität Bremen Prof. Dr. Alfred Schmidt, Universität Bremen Prof. Dr. Emily King, Universität Bremen Prof. Dr. Jens Rademacher, Universität Bremen Prof. Dr. Dmitry Feichtner-Kozlov, Universität Bremen Dr. Iwona Piotrowska-Kurczewski, Universität Bremen |
Time period: | 01.10.2016 - 31.03.2021 |
Website: | https://www.uni-bremen.de/rtg-pi3 |
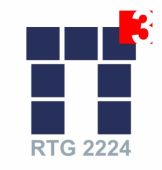
Publications
- M. Beckmann, N. Heilenkötter.
Equivariant Neural Networks for Indirect Measurements.
SIAM Journal on Mathematics of Data Science, 6(3), 2024.DOI: 10.1137/23M1582862
online at: https://epubs.siam.org/doi/10.1137/23M1582862 - M. Beckmann, A. Bhandari, M. Iske.
Fourier-Domain Inversion for the Modulo Radon Transform.
IEEE Transactions on Computational Imaging, 10, 2024.online at: https://ieeexplore.ieee.org/stamp/stamp.jsp?arnumber=10499837
- F. Altenkrüger, A. Denker, P. Hagemann, P. Maaß, G. Steidl.
PatchNR: Learning from Very Few Images by Patch Normalizing Flow Regularization.
Inverse Problems, 39(6), 2023.online at: https://iopscience.iop.org/article/10.1088/1361-6420/acce5e/meta
- C. Arndt, A. Denker, S. Dittmer, J. Leuschner, J. Nickel, M. Schmidt.
Model-based deep learning approaches to the Helsinki Tomography Challenge 2022.
Applied Mathematics for Modern Challenges, 1(2), 2023.DOI: 10.3934/ammc.2023007
- A. Denker, I. Singh, R. Barbano, Z. Kereta, B. Jin, K. Thielemans, P. Maaß, S. Arridge.
Score-Based Generative Models for PET Image Reconstruction.
Erscheint in Machine Learning for Biomedical Imagingonline at: https://arxiv.org/abs/2308.14190
- S. Arridge, P. Fernsel, A. Hauptmann.
Joint Reconstruction and Low-Rank Decomposition for Dynamic Inverse Problems.
Inverse Problems and Imaging, 16(3):483-523, 2022.DOI: 10.3934/ipi.2021059
- P. Fernsel, P. Maaß.
Regularized Orthogonal Nonnegative Matrix Factorization and K-means Clustering.
Zur Veröffentlichung eingereicht.online at: https://arxiv.org/abs/2112.07641
- P. Fernsel.
Spatially Coherent Clustering Based on Orthogonal Nonnegative Matrix Factorization.
MDPI Journal of Imaging, 7(10), 2021.DOI: 10.3390/jimaging7100194
online at: https://www.mdpi.com/2313-433X/7/10/194 - S. Dittmer, T. Kluth, P. Maaß, D. Otero Baguer.
Regularization by architecture: A deep prior approach for inverse problems.
Journal of Mathematical Imaging and Vision, 62(3):456-470, Springer Verlag, 2020.DOI: 10.1007/s10851-019-00923-x
online at: http://link.springer.com/article/10.1007/s10851-019-00923-x - J. Clemens, T. Kluth, T. Reineking.
β - SLAM: Simultaneous Localization an Grid Mapping with Beta Distributions.
Information Fusion, 52:62-75, Elsevier, 2019. - A. Konschin, A. Lechleiter.
Reconstruction of a Local Perturbation in Inhomogeneous Periodic Layers from Partial Near Field Measurements.
Inverse Problems, 35(11), 114006, IOPscience, 2019.DOI: 10.1088/1361-6420/ab1c66
online at: https://iopscience.iop.org/article/10.1088/1361-6420/ab1c66 - J. Jacobsen, J. Behrmann, R. Zemel, M. Bethge.
Excessive Invariance Causes Adversarial Vulnerability.
International Conference on Learning Representations (ICLR), 2019.
online at: https://openreview.net/forum?id=BkfbpsAcF7
- J. Jacobsen, J. Behrmann, N. Carlini, F. Tramer, N. Papernot.
Exploiting Excessive Invariance caused by Norm-Bounded Adversarial Robustness.
SafeML Workshop, ICLR, 2019.
online at: https://arxiv.org/abs/1903.10484
- S. Dittmer, E. King, P. Maaß.
Singular values for ReLU layers.
IEEE Transactions on Neural Networks and Learning Systems, Article , 2019.online at: https://ieeexplore.ieee.org/document/8891761
- T. Kluth, B. Jin, G. Li.
On the Degree of Ill-Posedness of Multi-Dimensional Magnetic Particle Imaging.
Inverse Problems, 34(9), 2018. - D. Otero Baguer, P. Maaß.
Inverse Problems in designing new structural materials.
7th International Conference on High Performance Scientific Computing, 19.03-23.03.2018, Hanoi, Vietnam.
- J. Leuschner, M. Schmidt, P. Fernsel, D. Lachmund, T. Boskamp, P. Maaß.
Supervised Non-negative Matrix Factorization Methods for MALDI Imaging Applications.
Bioinformatics, bty909 , 2018. - C. Meerpohl, K. Flaßkamp, C. Büskens.
Optimization Strategies for Real-Time Control of an Autonomous Melting Probe.
2018 American Control Conference (ACC), 2018, Milwaukee, WI, USA.
- K. Schäfer, M. Runge, K. Flaßkamp, C. Büskens.
Parameter Identification for Dynamical Systems Using Optimal Control Techniques.
European Control Conference (ECC) 2018, 12.06.-15.06.2018, Limassol, Cyprus.
- W. Heins, C. Büskens.
Two-Level Forecast-Based Energy and Load Management for Grid-Connected Local Systems Using General Load and Storage Models.
18th International Conference on Environment and Electrical Engineering (EEEIC), 12.06-15.06.2018, , Italy.
- P. Fernsel, P. Maaß.
A Survey on Surrogate Approaches to Non-negative Matrix Factorization.
Vietnam Journal of Mathematics, 46(4):987-1021, Springer Verlag, 2018. - J. Behrmann, S. Dittmer, P. Fernsel, P. Maaß.
Analysis of Invariance and Robustness via Invertibility of ReLU-Networks.
Zur Veröffentlichung eingereicht.online at: https://arxiv.org/abs/1806.09730
- J. Behrmann, C. Etmann, T. Boskamp, R. Casadonte, J. Kriegsmann, P. Maaß.
Deep Learning for Tumor Classification in Imaging Mass Spectrometry.
Bioinformatics, 34(7):1215-1223, Oxford University Press, 2018. - T. Kluth.
Mathematical models for magnetic particle imaging.
Inverse Problems, 34(8), 2018. - J. Clemens, C. Meerpohl, V. Schwarting, M. Rick, K. Schill, C. Büskens.
Autonomous In-Ice Exploration of the Saturnian Moon Enceladus.
69th International Astronautical Congress (IAC), 01.10.-05.10.2018, Bremen, Germany.
- C. Bathke, T. Kluth, C. Brandt, P. Maaß.
Improved image reconstruction in magnetic particle imaging using structural a priori information.
International Journal on Magnetic Particle Imaging, Article ID 1703015, 3(1), 10 pages, 2017. - T. Kluth, P. Maaß.
Model uncertainty in magnetic particle imaging: Nonlinear problem formulation and model-based sparse reconstruction.
International Journal on Magnetic Particle Imaging, Article ID 1707004 3(2), 10 pages, 2017. - T. Gerken, A. Lechleiter.
Reconstruction of a Time-dependent Potential from Wave Measurements.
Inverse Problems, Article ID 094001 33(9), IOPscience, 2017.Ausgezeichnet als Highlight Paper
DOI: 10.1088/1361-6420/aa7e07
online at: http://iopscience.iop.org/article/10.1088/1361-6420/aa7e07