EU-UNLocX: Uncertainty principles versus localization properties, function systems for efficient coding schemes
Working Group: | WG Industrial Mathematics |
Leadership: | Prof. Dr. Dr. h.c. Peter Maaß ((0421) 218-63801, E-Mail: pmaass@math.uni-bremen.de ) |
Processor: |
Dr. Jan Hendrik Kobarg
Sabine Eifeld (E-Mail: eifeld@math.uni-bremen.de ) |
Funding: | Europäische Kommission |
Project partner: |
Prof. Pierre Vandergheynst, EPFL Prof. Bruno Torrésani, Université de Provence Nir Sochen, Tel Aviv University Hans-Georg Stark, Hochschule Aschaffenburg Prof. Hans Georg Feichtinger, Universität Wien SagivTech Ltd. Steinbeis Innovationszentrum SCiLS, Steinbeis Innovation gGmbH Genesis S.A. European Research Services GmbH |
Time period: | 01.09.2010 - 31.08.2013 |
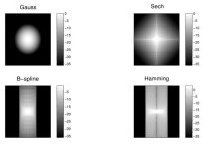
Algorithms in signal and image processing have reached an impressive level of
sophistication and computing power still increases at an exponential rate. However, high-
tech applications have an ever-increasing demand for even more efficient algorithms, even
more powerful computers and new concepts for advancing applications.
Starting from a recently discovered gap in the theory of uncertainty principles, this project
aims at developing a framework for constructing problem adapted, ultra-efficient algorithms
concerning (de-)coding and analyzing/synthesizing signals/images. We expect, that this will
allow us to tackle complex applications in life sciences and ultra precise audio signal
processing which presently cannot be solved appropriately with existing algorithms on
existing computers.
The key for developing these algorithms is a representation of signals and images by
function systems, which satisfy the following requirements:
1. Optimal localization,
2. Efficient discretization.
The theoretical foundation of this approach is based on the definition of suitable localization
measures in generalized phase spaces and the construction of minimizing waveforms. These
waveforms are then the basic building blocks in discretization schemes.
We expect that this approach allows us to shift the limits of the efficiency vs. precision
paradigm considerably. The efficiency of an abstract algorithm has to be evaluated in
connection with the computer hardware (parallelization, data exchange, storage) used.
Accordingly, our proof of principle includes implementations of baseline algorithms as well as
of advanced GPU implementations.
As final proof of principle we apply these methods for two challenging applications in audio
signal design and life sciences (proteomics). The evaluation will be done by our industrial
consortium partners together with our advisory board consisting of one SME, one world
market leader and two internationally highly recognized scientific experts.
Publications
- J. H. Kobarg, P. Maaß, J. Oetjen, O. Tropp, E. Hirsch, C. Sagiv, M. Goldabaee, P. Vandergheynst.
Numerical experiments with MALDI Imaging data.
Advances in Computational Mathematics, 40(3):667-682, 2014. - D. Trede, S. Schiffler, M. Becker, S. Wirtz, K. Steinhorst, J. Strehlow, M. Aichler, J. H. Kobarg, J. Oetjen, A. Dyatlov, S. Heldmann, A. Walch, H. Thiele, P. Maaß, F. Alexandrov.
Exploring Three-Dimensional Matrix-Assisted Laser Desorption/Ionization Imaging Mass Spectrometry Data: Three-Dimensional Spatial Segmentation of Mouse Kidney.
Analytical Chemistry, 84(14):6079-6087, 2012.DOI: 10.1021/ac300673y
- D. Trede, F. Alexandrov, C. Sagiv, P. Maaß.
Magnification of Label Maps with a Topology-Preserving Level-Set Method.
IEEE Transactions on Image Processing, 21(9):4040-4053 , 2012. - F. Alexandrov, S. Meding, D. Trede, J. H. Kobarg, B. Balluff, A. Walch, H. Thiele, P. Maaß.
Super-resolution segmentation of imaging mass spectrometry data: Solving the issue of low lateral resolution.
Journal of Proteomics, 75(1):237-245, Elsevier, 2011. - F. Alexandrov, J. H. Kobarg.
Efficient spatial segmentation of large imaging mass spectrometry datasets with spatially aware clustering.
Bioinformatics, 27(13):i230-i238, 2011. - D. Trede, J. H. Kobarg, K. Steinhorst, F. Alexandrov.
Mathematical Methods for Imaging Mass Spectrometry.
14th Joint International IMEKO TC1+TC7+TC13 Symposium, 31.08.-02.09.2011, Jena, Germany.Best Paper Award at IMEKO Symposium Jena
online at: URN: urn:nbn:de:gbv:ilm1-2011imeko-082:8