Sparsity and Compressed Sensing in Inverse Probleme
Working Group: | WG Industrial Mathematics |
Leadership: | Prof. Dr. Dirk Lorenz ((0421) 218-63982, E-Mail: d.lorenz@uni-bremen.de ) |
Processor: |
Dr. Stefan Schiffler
Prof. Dr. Dirk Lorenz ((0421) 218-63982, E-Mail: d.lorenz@uni-bremen.de ) |
Funding: | DFG SPP1324 |
Project partner: | Prof. Dr. Gerd Teschke, Hochschule Neubrandenburg |
Time period: | 01.06.2008 - 31.05.2011 |
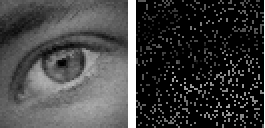
This project aims at a theory of compressed sensing for ill-posed problems and is hence located at the intersection of signal processing and ill-posed problems. Compressed sensing is a promising new field which tries to tackle the problem of high-dimensional data by combining the measuring and the compression step into one single process of "compressive sampling''. The theory is well developed for well-posed finite dimensional linear problems. Since in many applications it is not possible to measure the data directly but only a degraded version of the data by an ill-posed operator, there is need for a formulation for ill-posed problems in infinite dimensional spaces.
The main points to be addressed in this project are a proper formulation in infinite dimensional spaces and the treatment of ill-posed operators (e.g. compact operators) - both linear and non-linear. A central step is to find a substitute for the restricted isometry property which ensures the perfect recovery property. Moreover, is has to be clarified in which sense perfect recovery can be obtained for ill-posed problems. The robustness with respect to noise - which parallels the convergence rates for inverse problems - is another point of investigation.
To make the theory applicable in practice, an efficient recovery algorithm is needed. We are going to investigate the recovery by $\ell^1$-constrained minimization (which is called basis pursuit in compressed sensing). This approach is promising since $\ell^1$-constrained minimization has proven to have regularizing properties. Current methods for $\ell^1$-constrained minimization usually are inefficient when applied to ill-posed problems. In this project we are going to investigate the recently proposed semismooth Newton method which has been proven to work for ill-posed problems in infinite dimensions. In the second phase of the project, other recovery algorithms like the orthogonal matching pursuit will be considered.