Combination of Wavelet Shrinkage and Regularisation Method to Solve Ill-Posed Inverse Problems
Working Group: | WG Industrial Mathematics |
Leadership: | Prof. Dr. Dr. h.c. Peter Maaß ((0421) 218-63801, E-Mail: pmaass@math.uni-bremen.de ) |
Processor: | Dr. Esther Klann |
Project partner: | |
Time period: | 01.10.2001 - 30.04.2006 |
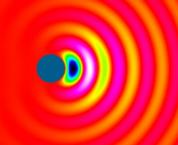
Ill-posed inverse problems are distinguished by a high sensibility towards errors in the input data. Classic regularisation methods such as the Tikhonov method use the aberrant data without prior data smoothing to solve the inverse problem. The theme of Esther Klann's dissertation project (supervised by Peter Maaß and Ronny Ramlau) is the analysis and application of coupled methods to solve inverse problems consisting of a data smoothing method, especially wavelet shrinkage, and a regularisation method. The combination of wavelet shrinkage and Tikhonov regularisation (TikShrink) has already been thoroughly investigated in the project to date. This has the underlying hypothesis of an operator smoothing in Sobolev and Besov scales as well as a stochastic error model. The wavelet shrinkage is carried out as hard shrinkage with a level-independent, global threshold value. A feasibility condition for the wavelet is given by the smoothing characteristic of the operator. For the Tikhonov regularisation performed subsequently an explicit parameter regulation was determined which, as a whole, leads to a quasi-optimal convergence rate for the combined method. This result was able to be expanded to all order-optimal regularisation methods. For the method with pre-smoothing and Tikhonov regularisation this results in a smaller regularisation parameter than without pre-smoothing. The data smoothing thus means that less regularisation is required to solve the inverse problem. This observation led to an expansion of the class of the method which, when combined with adequate data smoothing, provides an optimal convergence rate. Taken on their own, these methods are no longer regularisation methods, however. One example is the reduced Tikhonov method: here the Tikhonov filter is attenuated with a non-negative exponent of less than 0.5.
The TikShrink method was implemented and applied to simulated tomography data from SPECT analyses (SPECT - Single Photon Emission Computed Tomography). The objective here is the reconstruction of the density distribution of a radio-pharmaceutical material inside the body from sinogram data measured outside the body. The measured data are described mathematically with the help of the damped radon transformation. The computations performed confirm the convergence rate expected from theory.