KI-based documentation for midwives
Working Group: | WG Industrial Mathematics |
Leadership: | Dr. Iwona Piotrowska-Kurczewski |
Processor: | |
Funding: |
BAB Die Förderbank für Bremen und Bremerhaven, Gefördert durch die Europäische Union. |
Project partner: | Karen Güttler, atacama Blooms GmbH & Co. KG |
Time period: | 01.05.2020 - 28.02.2022 |
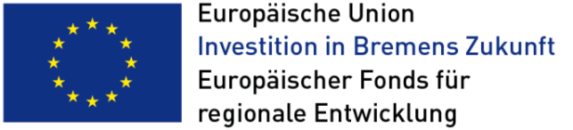
Although the number of midwives has increased slightly over the last ten years, more than half of them work part-time. The reasons for this are work overload, low remuneration and a high risk of regress. The latter has led to a sharp rise in liability insurance rates in recent years, with the result that fewer and fewer midwives are working freelance. Midwives employed in hospitals are relieved of liability insurance fees, but complain about a considerable high workload. KIDOHE aims to improve the midwives' stress and regress situation by means of an innovative, intelligent, decision-support system. The atacama blooms GmbH & Co. KG is planning to develop such a system in cooperation with the University of Bremen, which represents both scientifically founded expert knowledge and the experience of midwives in networks (e.g. semantic networks, Bayes networks or neural networks).
The technical work objectives are divided into two working areas: (1) The representation of expert knowledge in networks and (2) The linking of continuous data (CTG) with Boolean and categorical data (patient file, knowledge base) by means of neural networks (deep learning).