DELETO - Machine learning with correlative MR and high-throughput NanoCT
Working Group: | WG Industrial Mathematics |
Leadership: |
Dr. Tobias Kluth ((0421) 218-63817, E-Mail: tkluth@math.uni-bremen.de)
|
Processor: |
|
Funding: |
BMBF, Mathematik für Innovationen |
Project partner: |
Prof. Dr. Martin Burger, Friedrich-Alexander-Universität Erlangen
Prof. Dr. Thomas Schuster, Universität des Saarlandes
Siemens Healthineers, Erlangen, Siemens Healthcare GmbH
ProCon X-Ray GmbH, Sarstedt, ProCon X-Ray GmbH, Sarstedt
Radiologisches Institut, Universitätsklinikum Erlangen, Radiologisches Institut, UK Erlangen
|
Time period: |
01.04.2020 - 31.03.2023 |
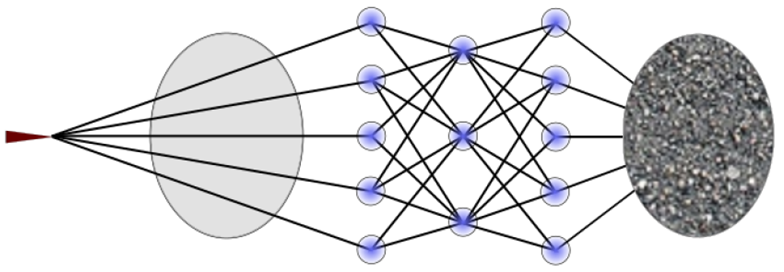
Magnetic resonance (MR) imaging is a widely used technique in medical imaging. Limitations are the long measurement time and the limited quantitative information associated with most MR techniques. To solve these problems, learning-based methods combined with model-driven approaches will be used. High-throughput nano-computer tomography (NanoCT) is based on transmission measurements of X-rays in the high-resolution range (up to 60 nm). In addition to the usual absorption imaging, phase and darkfield contrasts can be reconstructed. NanoCT allows the high-precision inspection of materials, such as aluminium alloys and fibre-reinforced plastics, which are of utmost economic importance (e.g. aircraft construction, automotive industry, wind turbines). Materials testing is of enormous importance in order to derive stress-strain relationships or to make statements about material failure. New requirements and materials demand ever higher resolutions with simultaneous high throughput. Sophisticated, novel mathematical methods are needed to overcome difficulties of current devices, such as inaccuracies in the measurement geometry, or to develop more efficient reconstruction algorithms for existing measurement data from the big data range.
Machine Learning (ML) and in particular the learning of large Neural Networks (NN), the so-called Deep Learning (DL), are currently among the most viral and widely discussed scientific topics, which have applications in many research areas. In the joint project DELETO, the mathematical research of DL in the solution of inverse problems is to be decisively advanced in order to make the reconstruction methods based on structural priorities and motion correction in the field of correlative MR and high-throughput NanoCT, which are computationally demanding due to the large amounts of data, more accurate and efficient. The goal is to integrate these methods into next generation devices. To this end, we are working closely with corresponding industrial partners. For the first time, model-based and data-driven methods will be applied in the big data technologies of correlative magnetic resonance imaging and high-throughput NanoCT, thus leading to improved next-generation devices.
The University of Bremen participates in DELETO with the sub-project "Invertable Residual Networks", which aims at theoretical investigations of the regulatory properties of certain network architectures, invertible residual networks (IRN).