AGENS - Analytical-generative Networks for System Identification
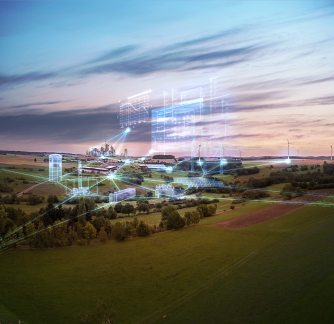
Forecasting the energy demand of individual actors on the basis of time series is characterized by an enormous amount of data due to the large number of energy consumers. Currently, every commercial customer with a consumption > 100 MWh per year is subjected to a recording power measurement and an individual consumption forecast is created for each customer. Flexible data-driven solutions are required to be able to map this complexity with mathematical models. On the one hand, this extensive data situation poses challenges for the handling of the analysis, but on the other hand, it also enables data-driven modelling of complex behaviour patterns. A core problem, however, lies in the transfer of this model to the individual prognosis for each actor.
The goal of AGENS is the development of flexible models based on neural networks (NN), which are able to model the overall complexity using large amounts of data. To enable a robust prognosis per actor, an improvement of the data quality for each individual consumer is necessary. As a core element of the project, so-called Generative Adversarial Neural Networks (GAN) are developed, which enable data augmentation. For a successful training of the GAN, statistical pre-analyses of the data have to be carried out in order to determine their characteristic patterns and feed them into the GAN. Based on the extended data set, the goal is to be able to train robust models with calibrated uncertainties and to ensure their applicability in an industrial setting.
The subproject "Dynamic Neural Networks" of the University of Bremen investigates the mathematical-theoretical relationships between time-recurrent neural networks (RNNs) based on discrete data (time series) and the continuous concepts of Neural Ordinary Differential Equations (NODE). The aim is to estimate the approximation quality under consideration of model uncertainties. These models will be tested in cooperation with industrial partners to predict the power demand. The main subject of this subproject is the development and analysis of dynamic neural networks with focus on energy prediction.