Neural networks in MALDI imaging
Working Group: | WG Industrial Mathematics |
Leadership: | Prof. Dr. Dr. h.c. Peter Maaß ((0421) 218-63801, E-Mail: pmaass@math.uni-bremen.de ) |
Processor: |
Dr. Jens Behrmann
Dr. Christian Etmann Dr. Tobias Boskamp Dr. Maximilian Schmidt ((0421) 218-63826, E-Mail: maximilian.schmidt@uni-bremen.de) |
Funding: | DFG-Graduiertenkolleg π³ |
Project partner: | |
Time period: | since 01.10.2016 |
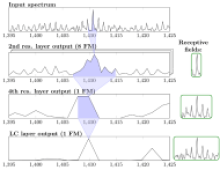
Figure: Processing of mass spectra via a neural network. Raw mass spectra serve as input at the top, which are progressively transformed by the layers of the networks to finally allow for a discrimination between different types of tissue tumor in the last layer.
Artificial neural networks were originally inspired by the information processing capabilities of the human brain, in order to uncover complex dependencies in massive data sets. During the course of the last years deep neural networks, a paradigm known as Deep Learning, have revolutionized areas like image and speech recognition. This incredible success is grounded in the enormous flexibility of these complex models, which are able to adapt its inner workings to the data at hand.
In contrast to these fields, neural networks have not yet made its entry to the classification of MALDI-Imaging spectra. Thus, a part of this project is to study how the architectures of neural networks have to be adapted to the processing of mass spectra in order to classify tumor in tissue. This application is particularly characterized by highly varying data characteristics due to the biological diversity of tissue tumor. Furthermore, the measurement process exhibits high uncertainties due to complex chemical and physical interactions. Despite those challenges, modified convolutional neural networks have shown first promising results.
A further main focus is the development of robust training methods to account for the mentioned high diversity. Especially this aspect is challenging as a potential application of automatic classification of tissue tumor to a diagnostic routine requires high accuracy.
Besides the development of robust and adaptive methods, interpretability of neural networks is a major concern for the application in medical decision building. Therefore, this projects aim at uncovering the inner workings of these often as black-box regarded models. First results showed how entirely data-based trained neural networks responded automatically to known biomarkers from histopathology.