Torelance-based regularization theory for inverse problems
Working Group: | WG Industrial Mathematics |
Leadership: | Prof. Dr. Dr. h.c. Peter Maaß ((0421) 218-63801, E-Mail: pmaass@math.uni-bremen.de ) |
Processor: |
Dr. Phil Gralla
Dr. Iwona Piotrowska-Kurczewski |
Project partner: | LFM, Labor für Mikrozerspanung |
Time period: | since 01.06.2015 |
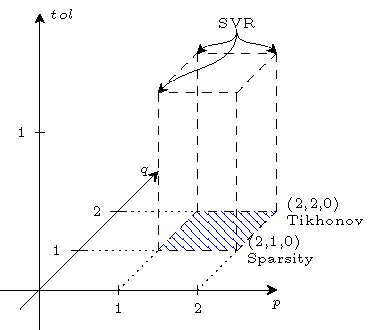
Tikhonov functionals for solving inverse problems consist of a discrepancy and a penalty term. The discrepancy term evaluates the deviation of the operator evaluation from the measured data. We propose to adjust the discrepancy term by incorporating a tolerance measure, which neglects deviations from the data within a prescribed tolerance. This is motivated e.g. by applications where multiple measurements of the same object are available. Thus providing a confidence area for the true data. This approach is known for the identity operator and used in support vector regression.
Regularization theory for Tikhonov functionals
with F:U → V and fixed p,q is well established. The research focuses on building a mathematical framework for tolerances in the discrepancy term of Tikhonov functionals (1) for a non-linear operator F : U → V and noisy data v δ . At first a general definition of tolerance and tolerance area is needed for elements of Hilbert Spaces. This general definition needs to include the known cases of support vector regression and its extensions. Based on this definition a mathematical frame work including existence of minimizer, stability and convergence rates for p, q, ε is derived. This work will be the main focus currently, with an extension for numerical solutions including algorithms and their implementation.
Different eyamples, taken mostly from the cooperation with the Collaborative Research Center 747 (SFB 747), illustrate the advantages of our approach compared to existing methods. One main example is parameter identification for micro milling processes. Micro milling processes face new challenges due to size effects and demanded high manufacturing precision. Our mathematical background helps to develop tools and methods to support engineers with their task. One of these is producing surfaces with predefined characteristics through micro milling. The surface is highly depended on process parameters such as cutting depth and feeding speed. Identifying the right parameter set can be done using methods from inverse problems such as the proposed altered Tikhonov functional.