Biomarker discovery in MALDI-TOF mass spectrometry with discrete wavelet transformation
Working Group: | WG Industrial Mathematics |
Leadership: | Dr. Fedor Alexandrov |
Processor: | Dr. Fedor Alexandrov |
Project partner: |
Prof. Dr. Herbert Thiele, Bruker Daltonik GmbH, Bremen Prof. Dr. Andre M. Deelder, Leiden University Medical Center, the Netherlands |
Time period: | 15.02.2007 - 31.03.2010 |
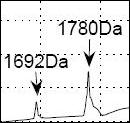
This main aim of this joint project involving a collaboration of the Center for Technomathemat-ics, the major mass-spectrometry instruments provider Bruker Daltonik and the academic health science center Leiden University Medical Center is the development of a procedure for bio-marker discovery in mass spectrometry (of the MALDI-TOF type) protein profiles using discrete wavelet transformaition and support vector machines. We are working with the colorectal cancer and schistosomiasis disease data collected in the LUMC and measured with Bruker mass-spectrometers.
The use of mass spectrometry for searching the biomarkers (usually peptides correlating with the infection level) in cancer diagnostics was suggested by Petricoin et al. (2002) factually flawed. The second-generation studies discovered the importance of avoiding bias and overfitting as well as the need of validation (Ransohoff, 2004).
The general scheme of finding putative biomarkers is as follows. First, the mass spectra are to be preprocessed. Then, they can be coded providing an alternative data representation and we used discrete wavelet transformation (DWT) for this aim. Next, a feature selection or a feature extrac-tion can be optionally applied. The classification of the data following the supervised learning approach is performed. If the classifiers produced allow to discriminate the infected and non-infected samples (spectra) then one can interpret the information used in the classification in terms of the peaks presented in the spectra. Finally, using another round of measurements of the same samples with higher resolution, the peptides can be associated with the peaks.
In this project we are planning to perform all the steps of biomarker discovery combining dis-crete wavelet transformation, statistical methods of feature selection and support vector ma-chines technique. We pay special attention to generalizability of the achieved results or robust-ness to variability influenced not by the disease but by the day of measurements, the laboratory used, peculiar properties of the individuals (age etc.). The general properties of the produced classifiers are to be studied using the support vectors properties as well as in a direct way doping noise or spurious peaks to the spectra classified.