Team for Deep Learning & Digital Pathology (DigiPath)
The digipath team tackles different challenges in the area of digital pathology, for example the classification of tumor and non-tumor tissue or of different tumor types based on digital microscopy images. To that end, classical machine learning as well as state-of-the-art deep learning methods are used.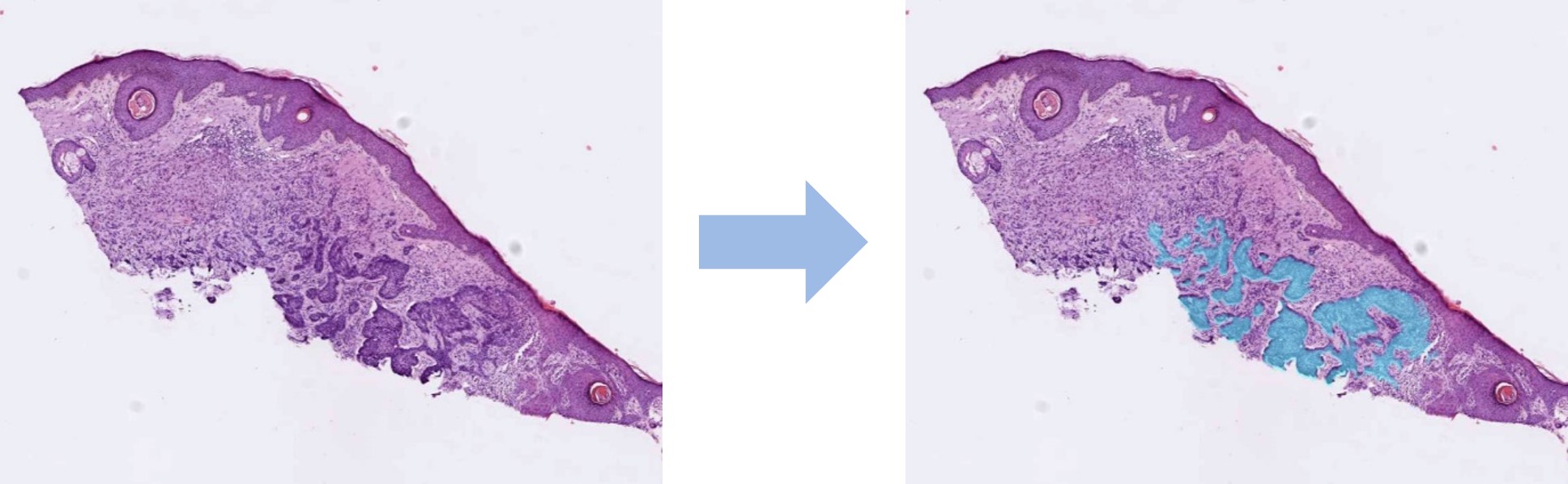
Projects
- SPAplus: Small data problems in digital pathology and measures accompanying the programme
- DIAMANT - Digital Image Analysis and Imaging Mass Spectrometry to Differentiate Non-small Cell Lung Cancer
- MALDISTAR - Study on quality assessment, standardization and reproducibility of data from MALDI imaging mass spectrometry
DigiPath - Viewer
Additionally, the team is developing a „DigiPath-Viewer“, a web application that can be used to easily annotate digital microscopy images and to view the results of the classification models. The „DigiPath-Viewer“ is intended to visualize our results and also to facilitate the work of patholgists. Some of the main features are:- Integration with stylus pen for natural and precise annotations.
- A semi-automatic annotation tool that speeds up the annotation process. The annotation polygon can be easily adjusted by dragging/erasing the points.
- Visualization of artificial intelligence automatic tumor predictions as overlays on the original images. The overlay's transparency as well as the threshold to differentiate between tumor or normal tissue can be easily adjusted.
Participation in challenges
- CAMELYON17: The goal of this challenge is the development of a method for the automated detection and classification of breast cancer metastases in whole-slide images of histological lymph node sections. This task would normally require extensive microscopic evaluation by pathologists. Therefore, an automated solution would be very promising to reduce the workload of pathologists and at the same time reduce the subjectivity in diagnosis. Our team obtained the 13th place in the unofficial leaderboard, which has remained open after oficial deadlines and has received more than 100 submissions from the best digital pathology companies and research groups.
Team leader
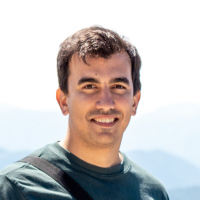
The team
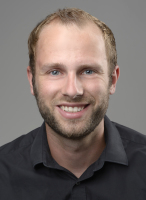
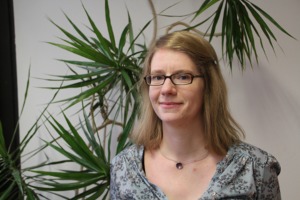
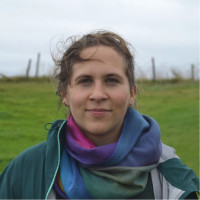
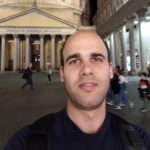
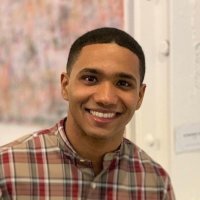
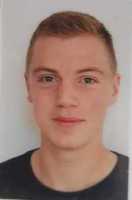
Publications
- J. Behrmann, C. Etmann, T. Boskamp, R. Casadonte, J. Kriegsmann, P. Maaß.
Deep Learning for Tumor Classification in Imaging Mass Spectrometry.
Bioinformatics, 34(7):1215-1223, Oxford University Press, 2018. - C. Etmann, M. Schmidt, J. Behrmann, T. Boskamp, L. Hauberg-Lotte, A. Peter, R. Casadonte, J. Kriegsmann, P. Maaß.
Deep Relevance Regularization: Interpretable and Robust Tumor Typing of Imaging Mass Spectrometry Data.
Zur Veröffentlichung eingereicht.online at: https://arxiv.org/abs/1912.05459