OPA³L - Optimally Assisted, Highly Automated, Autonomous and Cooperative Vehicle Navigation and Localization
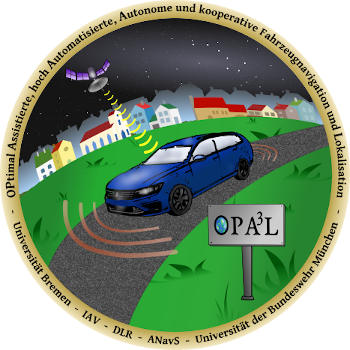
The hybrid vehicle used in the project enables an effective and environmentally friendly choice between electric and conventional propulsion. Furthermore, the research car is equipped with a multitude of sensors, such as cameras, radar, ultrasonic and laser scanners, in order to be able to act autonomously. With these sensor data, the car can recognize its surroundings. A computer-generated image of the real test vehicle and the recognized environment then allows the implementation of optimal, fast, comfortable, safe and fuel-efficient driving maneuvers.
The project is coordinated by the Working Group for Optimization and Optimal Control of the Center for Industrial Mathematics and carried out in close cooperation with the Working Group for Cognitive Neuroinformatics. Further partners in the project are the Computer Graphics and Virtual Reality Working Group of the University of Bremen and the Institute for Space Technology and Utilisation of Space at the Bundeswehr University Munich, as well as the industrial companies IAV and ANavS.
Publications
- A. Folkers, C. Wellhausen, M. Rick, X. Li, L. Evers, V. Schwarting, J. Clemens, P. Dittmann, M. Shubbak, T. Bustert, G. Zachmann, K. Schill, C. Büskens.
The OPA3L System and Testconcept for Urban Autonomous Driving.
25th IEEE International Conference on Intelligent Transportation Systems, 08.10.-12.10.2022.
DOI: 10.1109/ITSC55140.2022.9922416
online at: https://ieeexplore.ieee.org/document/9922416 - A. Folkers, M. Rick, C. Büskens.
Time-Dependent Hybrid-State A⁎ and Optimal Control for Autonomous Vehicles in Arbitrary and Dynamic Environments.
21th IFAC World Congress, 11.07.-17.07.2020, Berlin, Germany.
Proceedings of the 21th IFAC World Congress, 53(2):15077-15083, 2020.DOI: 10.1016/j.ifacol.2020.12.2029
online at: https://www.sciencedirect.com/science/article/pii/S2405896320326653 - A. Folkers, M. Rick, C. Büskens.
Controlling an Autonomous Vehicle with Deep Reinforcement Learning.
Intelligent Vehicles Symposium, 09.06.-12.06.2019, Paris, France.
Proceedings of the 30th IEEE Intelligent Vehicles Symposium, pp. 2025-2031, 2019.Best Student Paper
- M. Rick, J. Clemens, L. Sommer, A. Folkers, K. Schill, C. Büskens.
Autonomous Driving Based on Nonlinear Model Predictive Control and Multi-Sensor Fusion.
10th IFAC Symposium on Intelligent Autonomous Vehicles (IAV 2019), 03.07.-05.07.2019.
- A. Folkers.
Steuerung eines autonomen Fahrzeugs durch Deep Reinforcement Learning.
BestMasters, 75 pages, Springer Verlag, 2019.