BMBF-MaDiPath: Mass spectrometric profiling and grading for oncologic routine applications in pathology
Working Group: | WG Industrial Mathematics |
Leadership: | Prof. Dr. Dr. h.c. Peter Maaß ((0421) 218-63801, E-Mail: pmaass@math.uni-bremen.de ) |
Processor: |
Dr. Jens Behrmann
Dr. Tobias Boskamp Dr. Yovany Cordero Hernandez Dr. Christian Etmann Dr. Lena Hauberg-Lotte Delf Lachmund Dr. Janina Oetjen Sabine Eifeld (E-Mail: eifeld@math.uni-bremen.de ) |
Funding: | BMBF, KMU-innovativ: Medizintechnik |
Project partner: |
Proteopath GmbH SCiLS, Zweigniederlassung der Bruker Daltonik GmbH |
Time period: | 01.10.2015 - 30.09.2018 |
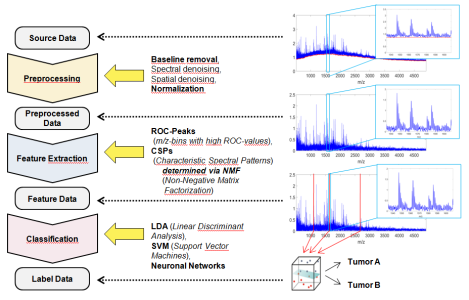
The joint research project MaDiPath deals with the exploration and establishment of mass spectrometric methods for digital pathology in the field of MALDI imaging. The goal of the project is the development of methods that allow an objective, reproducible and automated basis for pathological tumor diagnostic and for a corresponding patient-oriented therapy plan.
The used approach is based on proteomic relevant and a priori identified patterns of breast and ovarian carcinoma. These tumors are of big social and medical relevance: Breast cancer is the most frequent kind of cancer for women in Germany. Though the incidence rate is decreasing for ovary cancer, the recurrence rate is high and the chance for healing is low for the women concerned.
The sub-project of ZeTeM targets the development of an NMF method to determine the characteristic spectral peptide patterns for the use in classification and regression models of tumors from MALDI imaging data. The project follows a knowledge based approach where spectral patterns for known tumor markers are modeled and used as initial point for a data specific feature extraction (targeted feature extraction).
Blind source separation with Sparsity is of special meaning for mathematical research: Blind source separation is a general method to separate data generated by an overlap of different structures. These methods are combined with Sparsity approaches, i.e. among all possible overlaps approximating the data with sufficient accuracy; solutions with the fewest coefficients are preferred. For this, structures with small amplitudes, which actually lie below the noise levels of the measured data, can though be separated reliably. Transferred to imaging mass spectrometry this allows the description of very complex single spectra as overlap of only few spectral basis patterns.
Publications
- J. Leuschner, M. Schmidt, P. Fernsel, D. Lachmund, T. Boskamp, P. Maaß.
Supervised Non-negative Matrix Factorization Methods for MALDI Imaging Applications.
Bioinformatics, bty909 , 2018. - Y. Cordero Hernandez, T. Boskamp, R. Casadonte, L. Hauberg-Lotte, J. Oetjen, D. Lachmund, A. Peter, D. Trede, K. Kriegsmann, M. Kriegsmann, J. Kriegsmann, P. Maaß.
Targeted Feature Extraction in MALDI Mass Spectrometry Imaging to Discriminate Proteomic Profiles of Breast and Ovarian Cancer.
Proteomics - Clinical Applications, 1700168 , Wiley, 2018. - J. Behrmann, C. Etmann, T. Boskamp, R. Casadonte, J. Kriegsmann, P. Maaß.
Deep Learning for Tumor Classification in Imaging Mass Spectrometry.
Bioinformatics, 34(7):1215-1223, Oxford University Press, 2018. - T. Boskamp, D. Lachmund, J. Oetjen, Y. Hernandez-Cordero, D. Trede, P. Maaß, R. Casadonte, J. Kriegsmann, A. Warth, H. Dienemann, W. Weichert, M. Kriegsmann.
A new classification method for MALDI imaging mass spectrometry data acquired on formalin-fixed paraffin-embedded tissue samples.
BBA - Proteins and Proteomics, , 2016.